Home > Agriculture > Agri Machinery & Tech > Agri Equipment > Machine Learning for Crop Yield Prediction Market
Machine Learning for Crop Yield Prediction Market Size
Machine Learning for Crop Yield Prediction Market was valued at USD 581 million in 2023 and is estimated to register a CAGR of over 26.5% between 2024 and 2032. Various factors such as enhanced data quality of satellite imagery and improved accuracy of machine learning technologies are driving the market growth.
High-resolution and multi-spectral satellite images and drones deliver detailed insights into crop health, soil conditions, and environmental factors. Data integration significantly improves the quality of inputs for Machine Learning (ML) models as it enhances accuracy and increases model robustness. Moreover, integrating advanced data sources can substantially improve outcomes across various sectors, particularly in agriculture.
For instance, in May 2023, NASA introduced a program that provides farmers with useful information generated from satellite images of Earth. This initiative employs advanced Machine Learning (ML) techniques to analyze high-resolution satellite data, delivering precise and timely information on crop health, soil conditions, and environmental factors. This development signifies a major advancement in Machine Learning for the Crop Yield Prediction market, emphasizing the emerging potential of integrating satellite technology with ML to enhance sustainable agricultural productivity and resilience.
Report Attributes | Details |
---|---|
Base Year: | 2023 |
Market Size in 2023: | USD 581 Million |
Forecast Period: | 2024 to 2032 |
Forecast Period 2024 to 2032 CAGR: | 26.5% |
2032 Value Projection: | USD 4.5 Billion |
Historical Data for: | 2021 - 2023 |
No. of Pages: | 240 |
Tables, Charts & Figures: | 310 |
Segments covered: | Component, Deployment Model, Farm Size, End User |
Growth Drivers: |
|
Pitfalls & Challenges: |
|
Moreover, agritech startups are driving innovation in the agriculture sector by developing and implementing advanced machine-learning algorithms for crop yield prediction. These startups leverage extensive datasets, including weather, soil, and crop health data, to create more accurate and reliable predictive models. With access to the latest technology and agility in adopting new machine learning techniques, they develop cutting-edge solutions that enhance the accuracy and efficiency of crop yield predictions. These advancements optimize agricultural processes and promote sustainable farming practices, ensuring food security and economic stability for farmers and communities worldwide.
The effectiveness of machine learning models for crop yield prediction is significantly compromised by limited data availability. The dependency on large and diverse datasets is crucial for developing accurate and reliable models. It leads to models with higher error rates and reduced generalization capabilities in case of data insufficiencies, ultimately affecting their performance. Overfitting is a common issue in such scenarios that results in models that excel in training data but fail to provide reliable predictions on new or unseen data. These challenges raise several concerns in the adoption of these technologies, potentially hindering their adoption and growth. However, the prominent market players are investing in data collection, and exploring alternative data sources to address these issues. Further, these players are focused on implementing techniques that enhance model robustness and accuracy, even with limited data, thus creating lucrative opportunities for market growth.
Machine Learning for Crop Yield Prediction Market Trends
The adoption of machine learning (ML) algorithms and artificial intelligence (AI) technologies in agriculture is growing among farmers and Agritech companies to enhance productivity and efficiency. ML models can analyze extensive datasets, including weather patterns, soil health, and crop conditions, to predict yields with greater accuracy. Moreover, governments across the globe are highly investing in R&D initiatives for AI and machine learning models. For instance, according to the World Economic Forum, the U.S. government has invested USD 200 million in AI technology for the agriculture industry across the region to integrate the supply chain and risk resilience visibility for farmers. The government aims to drive advancements in agriculture by funding research and innovation. These efforts focus on improving crop yield predictions, optimizing resource management, and addressing modern agricultural challenges. This financial commitment highlights the government's focus on leveraging cutting-edge technologies to transform the agricultural sector, ensuring its future resilience and efficiency.
Moreover, technological advancements in agriculture enable better decision-making, optimize resource use, and enhance crop management. This leads to higher yields and promotes sustainable agricultural practices. As these technologies continue to evolve, they are expected to play a crucial role in shaping the future of agriculture.
Machine Learning for Crop Yield Prediction Market Analysis
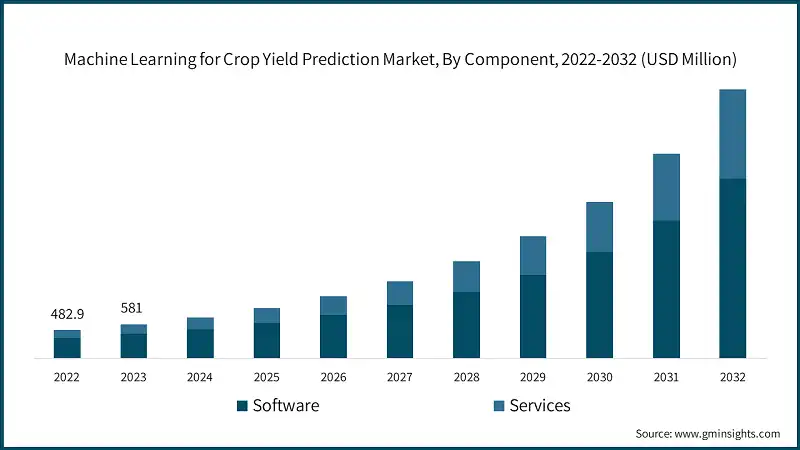
Based on component, the market is divided into software and services. In 2023, the software segment held approximately USD 413 million. These solutions are becoming increasingly crucial, due to their seamless integration with Internet of Things (IoT) devices and big data platforms. For instance, in November 2023, the Department of Agriculture and Farmers Welfare in collaboration with Wadhwani Institute for AI developed Krishi 24/7, the first AI-powered solution for automated agricultural news monitoring and analysis, has received support from Google.org. This platform addresses the need for an efficient mechanism to identify and manage agricultural news articles, aiding timely decision-making. These integrations enable real-time data collection and analysis, significantly enhancing the accuracy of yield predictions. The growing emphasis on precision agriculture is driving the demand for sophisticated software capable of analyzing complex datasets and providing actionable insights. This trend is driving software developers to develop more advanced and user-friendly solutions, thus creating lucrative opportunities for further market growth.
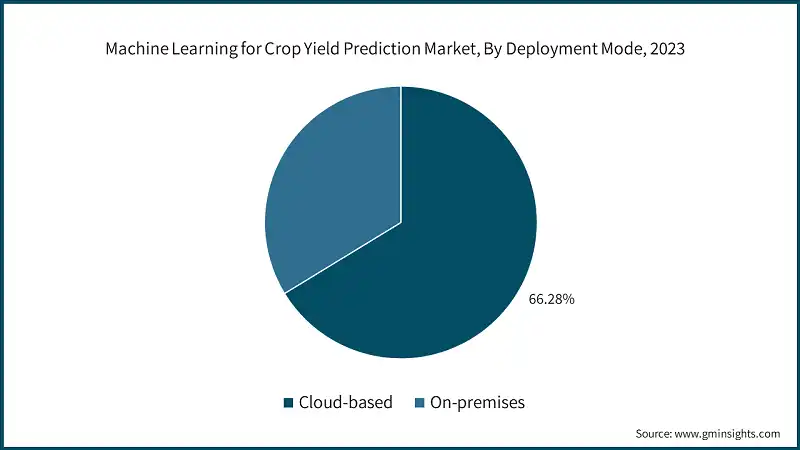
Based on the deployment model, the machine learning for crop yield prediction market is categorized into cloud-based and on-premises. The cloud-based segment is expected to hold over USD 3.2 billion by 2032. These platforms provide scalable resources, allowing users to adjust computing power and storage according to their needs. This flexibility is essential for managing large datasets and complex models in crop yield prediction. Moreover, cloud-based solutions eliminate the need for substantial upfront investments in hardware and infrastructure. Additionally, users can pay for the resources they use on a subscription or pay-as-you-go basis, making it a more economical option for many organizations Further, it offers easy access to ML tools and datasets from anywhere, facilitating collaboration among researchers, farmers, and Agritech companies. This accessibility streamlines workflows and enhances the sharing of insights and findings. Moreover, collaboration enables stakeholders to utilize their collective expertise and data, driving innovation and improving decision-making in the market.
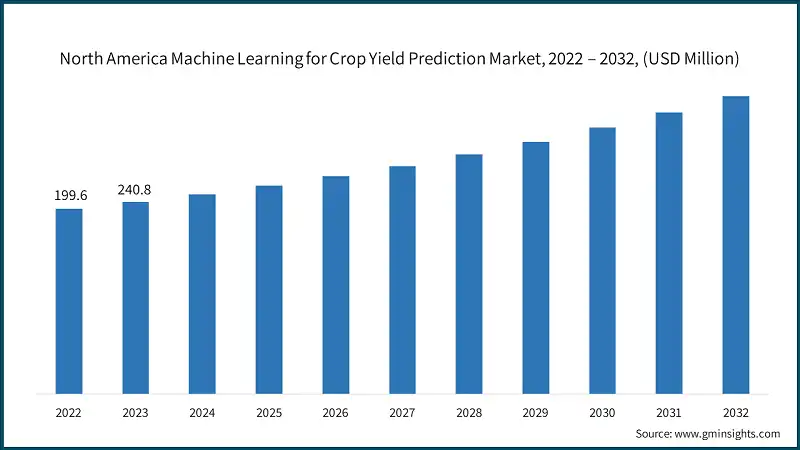
In 2023, North America dominated the machine learning for crop yield prediction market with around 41% of the market share. The region has a high-end repository of agricultural data, sourced from diverse channels such as satellite imagery, IoT sensors, and meteorological stations. This data availability plays a significant role in enhancing ML models, thereby enhancing the precision of yield forecasts. Additionally, both public and private companies from the U.S. have invested in AI and ML technology. These investments, spanning government grants and venture capital, are propelling the advancement of innovative technologies in agriculture across the region. Such financial capital catalyzes R&D activities and facilitates the implementation of cutting-edge solutions.
Moreover, governments across the Asia-Pacific region are actively promoting agricultural innovation through funding, subsidies, and policies designed to enhance productivity and sustainability. These efforts include significant investments in machine learning (ML) and artificial intelligence (AI) technologies. As a result, these initiatives are accelerating the adoption of advanced technologies in agriculture, promoting the development of more efficient and resilient farming practices. Furthermore, by leveraging ML and AI, the region is addressing its unique agricultural challenges, improving crop yields, and ensuring long-term food security and environmental sustainability.
The European Union (EU) has enacted policies and funding programs to modernize its agricultural sector. Initiatallowssuch as the Common Agricultural Policy (CAP) and Horizon Europe allow channeling of funds into cutting-edge technologies, such as machine learning (ML) for predicting crop yields. Through these investments, the EU seeks to boost agricultural productivity, bolster sustainability, and tackle various farming challenges. As a result, these efforts aim to foster innovation and empower the agricultural sector's resilience for the future.
Machine Learning for Crop Yield Prediction Market Share
Microsoft Azure and Corteva are the prominent players in the market holding approximately 17% of the market share. Microsoft Azure's cloud platform is designed for machine learning and data analytics, offering a comprehensive suite of tools and services. Azure Machine Learning, a central feature, allows users to build, train, and deploy ML models efficiently, significantly enhancing applications such as advanced crop yield predictions. It supports a wide range of AI and ML frameworks, including TensorFlow, PyTorch, and Scikit-Learn. This compatibility simplifies the development and deployment of complex ML models tailored for agricultural use.
Corteva prioritizes R&D investments to perfect ML models for predicting crop yields. By partnering with research institutions and adopting leading technologies, the company aims to enhance the precision and reliability of its predictive models. It integrates ML with advanced data analytics to process extensive agricultural datasets. These datasets include information from IoT sensors, satellite imagery, and field trials, providing farmers with more accurate forecasts and actionable insights.
Machine Learning for Crop Yield Prediction Market Companies
Major players operating in the market are:
- Ag Leader Technology
- Blue River Technology
- Corteva
- SAP
- Microsoft Azure
- Taranis
- Ceres Imaging
Machine Learning for Crop Yield Prediction Industry News
- In March 2023, Corteva Agrisciences introduced an advanced gene editing technology for corn hybrids. Utilizing machine learning data collected from farms, this early-stage concept integrates multiple disease-resistant traits into a single gene location using proprietary technology.
- In December 2023, John Deere invested USD 180 million to construct the Brazil Technology Development Center. With the establishment of the new Development Center, the company aims to reduce the development time for new solutions by up to 40%, depending on the project's type.
The machine learning for crop yield prediction market research report includes in-depth coverage of the industry with estimates & forecasts in terms of revenue (USD Billion) from 2021 to 2032, for the following segments:
Click here to Buy Section of this Report
Market, By Component
- Software
- Predictive modelling software
- Data analytics platform
- Others
- Services
- Professional
- Managed
Market, By Deployment model
- Cloud-based
- On-premises
Market, By Farm Size
- Small
- Medium
- Large
Market, By End User
- Farmers
- Agricultural cooperatives
- Research institutions
- Government agencies
- Others
The above information is provided for the following regions and countries:
- North America
- U.S.
- Canada
- Europe
- UK
- Germany
- France
- Italy
- Spain
- Russia
- Nordics
- Rest of Europe
- Asia Pacific
- China
- India
- Japan
- South Korea
- ANZ
- Southeast Asia
- Rest of Asia Pacific
- Latin America
- Brazil
- Mexico
- Argentina
- Rest of Latin America
- MEA
- South Africa
- Saudi Arabia
- UAE
- Rest of MEA
Frequently Asked Questions (FAQ) :